AI can help you manage customer across the lifecycle. Understanding the customers that have dropped off can often be a great way to boost sales and margin.
Use AI to re-activate customers
It is imperative we manage our customers that we have, the ones that we have gained or lost explicably or inexplicably and how do we scale that to make sure we capturing as much of the market that we are able. We ca use AI to re-activate customers.
It’s a complex world, one which is going to be totally different. The rules have changed and we probably need to get with the programme.
We’re going to help you understand what you should be aiming for and a process for getting there.
Uncertainty – more questions than answers
First thing to realise is that there is going to be a lot more data to understand.
You’ve got what you had before, you’ve got the changed state of play (+ve or -ve) and you have got what is going to happen.
You’re going to use what you used before, but how are you going to know what’s changed, with whom, why and what are you going to do about it.
Advanced analytics is one of the answers. The advantage that businesses that have advanced analytic capability will have over the next 12 months will start to become more and more apparent.
Already, those companies and brands are more comfortable with where they are at. This is because they already know they have the means to capitalise on any upside.Use AI to re-activate customers
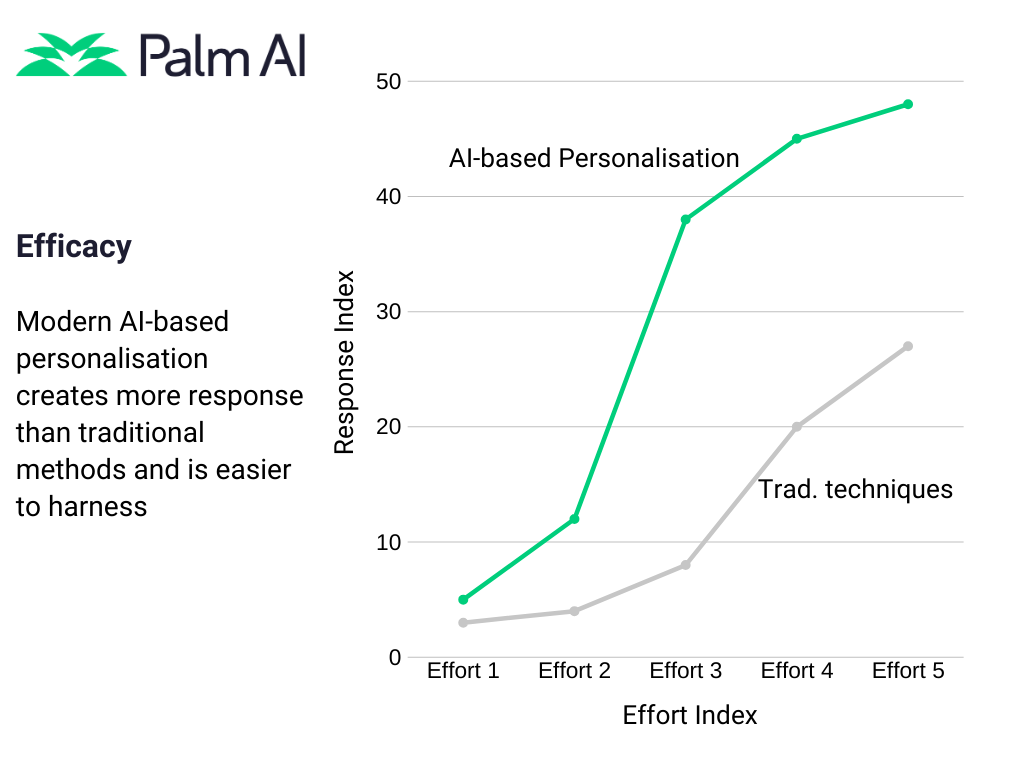
Faster time to deliver value
The chart to the right shows the difference in reponse rates compared to the effort between tradional BI (business intelligence) based personalisation and AI-based perso.
Customers now expect brands to be able to use personalisation and it still increases reposnse. Using initially quite obvious data points can show good returns quickly. Where you can get exponential growth in response is when you start to add extra datasets that are difficult to assimilate using BI techniques.
The added data sets, such as browsing data, social and external data sets are able to add the context and relevance that heightens the customer response. You are smashing it. Use AI to re-activate customers.
Faster process
Traditional techniques take a great deal of manual work to get right, check, proof and execute. With AI-based CRM you can automate many of those tasks, embed more insights and use those insights in your creative and messaging. That’s what drives the results.
Today our normal one-to-one process is as follows:
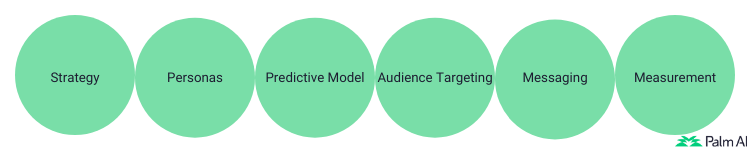
Traditional Process for Personalisation
So we start with some marketing objectives and strategy. We’re then going to use, tweak or create a persona or a few personas to help our targeting. We can then create a data brief to give us a predictive model that tells us that yes, we’re going to make some money and we’re going to change behaviour in an incremental way. We get that signed off and then we can use that model to do some audience targeting. Once we know the base we’re targeting we can then make sure the messaging is going to work and to keep it operationally simple we are probably going to keep the number of those offers and messages relatively few. This will help our measurement. Our measurement will probably then be done by an agency, maybe the same one that did the campaign…..and we will end with a deck of insights.
Problems with this methodology:
It’s a slow process
Insights & Results are not immediately actionable
Assumptions used for modelling are outdated and probably irrelevant
Faster learning, optimised response
We are better than that. Often brands won’t have all the data that they would like. There is a dark side when customers disappear from the journey, or the journey is just more complex than your simplified model. We therefore make assumptions, or use bridging techniques to make stuff work.
We know that there are many different types of customer all with different needs, they may be functional, they be aspirational, they may be event triggers.
To cover all the right messages and use the indexed or weighted data to decide tends to be a weighty process and only really ending up being relevant for the ones it is obvious to.
What this way of working is about is being able to use training data to work out what the optimised messaging is for each customer and then running that as an always on programme.
This isn’t about trying to find that one thing that is common to all. The answer is necessarily an X offer, or a Y service. It could be a blend of those at a distinct time, at the right location, with some other prevailing characteristic. The exciting part of this is that the model is transparent enough for you to see in, but powerful enough for you to know you’ve done the best job.
Each customer will get what they should get based on the data and your skill and art in crafting the message.
This takes you forward to right product, right time, right price, right channel.
Check out Databuilder here.
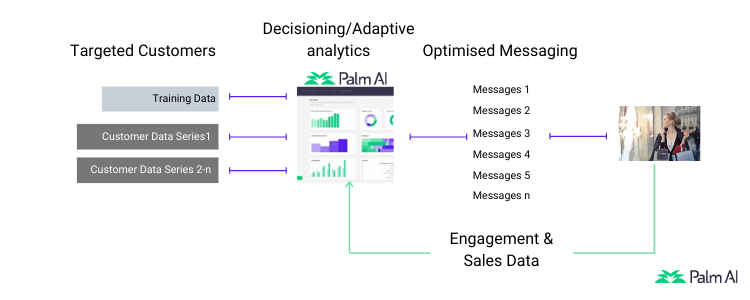
Conclusions
The speed that this happens can be immediate. The learnings are learnt systematically and applied to the current wave of the programme. Knowledge is kept within your brand as to what works and why. This is scaleable across your base. All messages are kept in play.
This is the reason that brands that use this approach are reporting such interesting results. It maybe that their most valuable customers feel valued, their ‘tail’ of customers is starting to wag, that their costs have halved, but their results are 20% up.
Or it may be that they have reactivated customers across a myriad of journeys because they didn’t have to plan it all out themselves. They had help. They had their AI.