Because of issues of privacy, regulation and accuracy, the use of first-party data is more and more important. Here we look at getting a plan to use it properly.
The importance of first-party data
Traditionally we code things to tell the machines to do exactly as we want them do. It’s detail and if it’s not in the brief, it probably won’t happen, if it’s not in the roadmap, it won’t happen. Even if it is, it might not happen.
We used try to find vendors that ticked all the boxes of what we wanted, compromised with some stuff depending on priorities, looked at the interface, costs, lifetime cost of ownership.
Those days are going to be over, thank goodness. The future is completely different and it’s going to start with customer and user needs. Using AI for core business activities will soon be as common as using databases is today. Why do I say that?
Seamless Experience
We now have more and more datasets available to us. Our businesses operate over a number of channels and customers expect a seamless experience across them
Convenience
Customers now expect us to service their requirements at a much higher level of convenience for them. They are also looking to do and experiment doing that that across many more touchpoints. Hence the rise of the voice assistant and the bots. Over 50% of teenagers use voice search every day
Need to grow share of wallet/voice/culture
AI can help with media buying, it can help with targeting, it can help with one-to-one personalisation across acquisition. What it is also very good at is understanding the potential for a customer over the lifecycle. Share of wallet, lifetime value, loyalty. It’s also part of how you grow your share of voice and even more than that, the culture of your industry or wider still. Because your insights are more scaleable and the data sources can be more diverse. If you have good data engineering that is.
First-party data
First party data is data that companies can collect themselves. From website, call centres, CRM, social media, transactions, surveys. You are already collecting it, you may not be optimising it for commercial benefit.
Importance of Data Engineering
Every business needs to have greater focus on it’s current base, it’s first party data. Privacy concerns, relationship concerns, customer expectations. All of these are meaning that brands now need to focus more than ever on their customers, their first-party data.
That means, at the very least, being able to pull your disparate data into one place. Create as much of a single customer view as you can. Understand your data points and what they mean. If your data is still a bit rubbish there will be a requirement to clean it and to change the source process so when you do clean it, you don’t keep polluting it.
This is where you need a data strategy, but also strong data engineering. Data engineering is how you transform data into a useful format for analysis. This is key to the success of your first project, decent data input. It is a critical, foundational function and one that we place the upmost importance on. It’s also one of the biggest problems for brands getting started. You can’t do AI without it, so let’s be clear on that, you need data engineering.
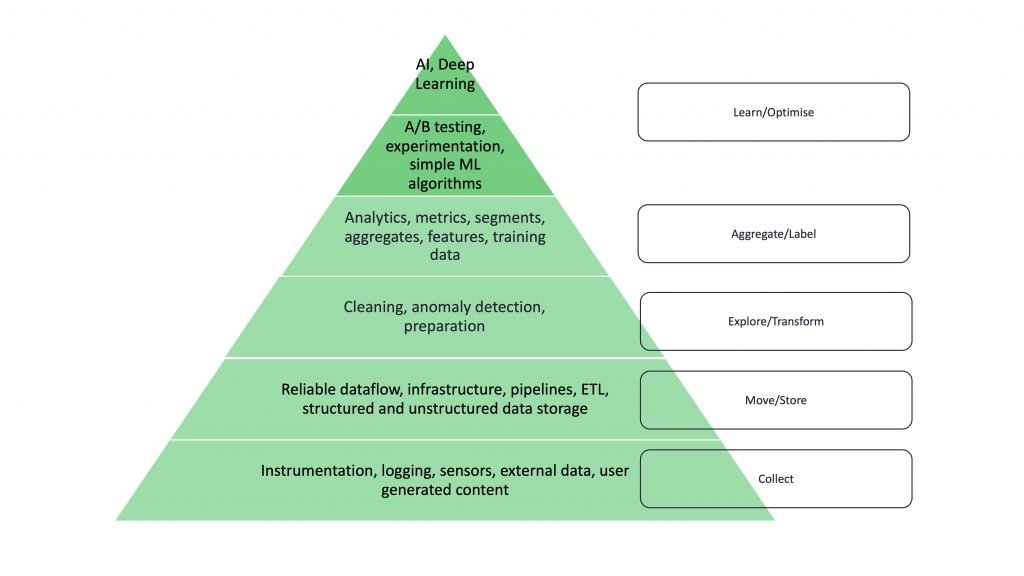
Hierarchy of Data Needs
I bet you didn’t know that there was a Data Science hierarchy of needs? Like Maslow (water, food, shelter, ipads, voice assistance), but for data. Here it is:
Thanks to Monica Rogati, appreciate the distil.
So, most brands will be doing the first 4 steps (from the bottom), to varying degrees of sophistication and requirement.
Typically, the whiz-bang BI tools sit in the aggregate and label box. Which give a level of visibility that you haven’t previously had.
The next stage is the intelligence. But you have to have the foundations in place. If not, you need to do the engineering to get that sorted.
Always Be Learning
he idea with an AI process, is being to be able to do the process from end -to-end. For a project to be valuable, to be successful and to be scaleable, you have to be able to see it through from the bottom to the top. That’s where you can stall, because the going can get tough.
Fear not, there is help for that. You can start small and grow, that’s another awesome bit. You don’t have to buy the Rolls Royce to start off, go small, be nimble, be agile, mitigate risk.
You will find because the end-to-end journey is not yet fully digitised, there will be data gaps. There are several ways to mitigate those gaps depending on the nature of the gap. It may be that a new digitisation process may be necessary, or that so-called synthetic data can be used. This is data that is created artificially in order to replicate statistical components of datasets, but without any identifiable information. Lookilikey data I guess.
There is a key difference with traditional systems that stop at the 4th level. They don’t learn.
The difference with AI is that it learns what’s required of it to deliver the outcome. You have stuff that you want to solve, traditional software, workflow software, won’t really help that much, it will give you the ‘mirage of solution’.
So the criteria has changed. Consider it more a journey, a path. Find your objectives, define your objectives.
It may be a recommendation engine, it may actually be more accurate billing to reduce customer frustration on bad admin.
It’s good to start with a problem that can show value to the business and be one of those ‘how are you going to solve that? It’s always been like that’ kind of things. Not that you want to set up to fail, so it’s finding a good balance between difficulty, value and colleague expectation.
Results – our favourite bit
One impactful project will create believers, in the boardroom, but also across other areas and especially with customers. You can look to solve problems that are common in the industry that will give you a competitive edge. Who’d have thought? Someone uses AI, data, process and creates a solution out of it, that benefits the business. It happens. ‘Hey, everyone’s billing is wrong, so we’re okay.’
Wrong. You that’s an immediate area for competitive advantage.
‘Hey, we’ve got 50% single line items and the industry norm is 55%, we’re okay.’
Wrong. Why is that your benchmark? How much is it worth to you to get that to 40%? 30%? About a zillion times more than the cost of doing it I bet.
Now you have the power to fix those problems. Now you can innovate. That’s why it’s good to start your journey now. The problems aren’t going away, your competitors are probably starting their journey and your chances of success have just doubled.
Your 4-point plan
Where is a pain for your customers and your business that hitherto has been too difficult/complex to solve but you have data for – i.e inaccurate invoicing.
Decide what you would like the outcome to be – i.e 95% reduction in invoicing queries.
Grab your data sets – i.e all invoices, all corrected invoices.
Start working up the pyramid. That’s it.