In today’s data-driven business landscape, making informed decisions based on comprehensive analysis is paramount. Here we look at how you can improve Business Intelligence using Artificial Intelligence. While traditional tools like Excel have served us well, they often fall short when dealing with large, complex datasets and multifaceted variables. This is where the power of artificial intelligence (AI) and algorithmic data processing shines, allowing businesses to unlock deeper insights and make more accurate predictions.
Traditional spreadsheet software like Excel is a valuable tool for basic data organization and analysis. However, as the volume and complexity of data grow, its limitations become increasingly apparent.
Here are three scenarios where Excel doesn’t make sense, and AI-powered solutions can provide a more robust and effective approach:
1. Predictive Maintenance in Manufacturing
In the manufacturing industry, unplanned equipment downtime can be extremely costly. Relying solely on Excel to track and analyze maintenance data may overlook critical patterns and relationships that could signal potential equipment failures. AI algorithms, on the other hand, can continuously monitor vast amounts of sensor data, identifying intricate correlations and anomalies that may indicate the need for preemptive maintenance, minimizing costly breakdowns and maximizing operational efficiency.
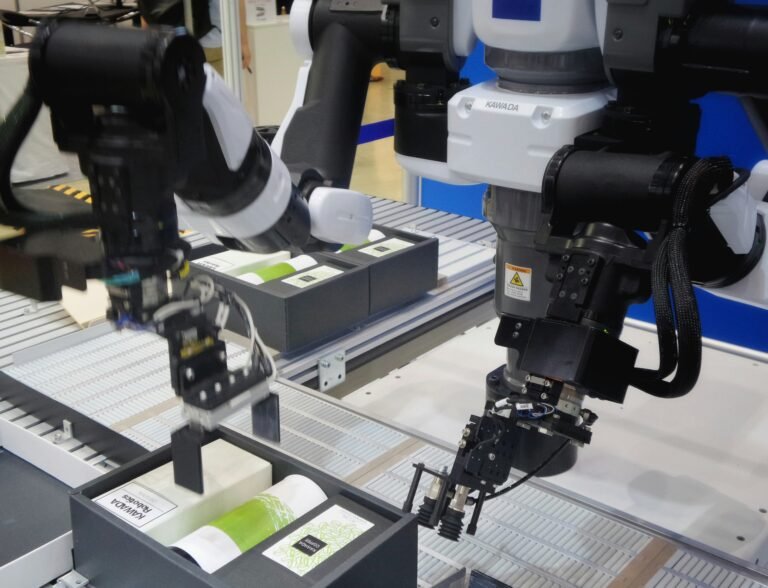
2. Customer Churn Analysis
Retaining customers is crucial for subscription-based businesses. While Excel can provide basic customer churn rates, it struggles to account for the multitude of factors that influence customer behaviour, such as usage patterns, demographic data, and customer feedback. AI models can ingest and analyze these diverse data sources, uncovering hidden patterns and predicting which customers are at risk of churning, enabling timely interventions and tailored retention strategies.
3. Fraud Detection in Financial Services
Financial institutions face the constant challenge of detecting and preventing fraudulent activities. Traditional rule-based systems and Excel-based analyses often fail to keep pace with the ever-evolving tactics of fraudsters. AI algorithms, however, can continuously learn from vast amounts of transactional data, identifying subtle patterns and anomalies that may indicate fraudulent behavior, allowing for more effective fraud prevention and mitigation.
The Real Power of AI
The power of AI lies in its ability to process and analyze vast amounts of data from multiple sources, accounting for intricate relationships and patterns that would be nearly impossible for humans to discern manually. This means you can improve Business Intelligence using Artificial Intelligence. Leveraging algorithmic data processing, businesses can gain a comprehensive understanding of their operations, customers, and market trends, enabling them to make more informed decisions, join up the execution throughout the business and stay ahead of the competition.
These three examples may help illustrate further.
1. Demand Forecasting for Retail and E-Commerce
Accurate demand forecasting is crucial for retailers and e-commerce businesses to optimize inventory levels, avoid stockouts or overstocking, and maximize profitability. While Excel can handle basic sales data analysis, it struggles to account for the numerous factors that influence consumer demand, such as seasonality, pricing, promotions, market trends, and consumer sentiment. Often specialist software that is based on time-series analysis struggles too. This means that Demand Forecasting professionals need to use their own intuition and stakeholder feedback to manipulate their forecasts. Sometimes these efforts negate the usefulness of the software.
AI algorithms, however, can ingest and analyze data from diverse sources, including historical sales data, pricing information, CRM and social media sentiment, competitor activity, PR and event-based influencers, demographic or geographic data. By identifying complex patterns and relationships, AI models can provide more accurate demand forecasts, enabling businesses to make informed decisions about inventory management, production planning, and marketing strategies.
2. Dynamic Pricing Optimization
Determining the optimal pricing strategy is a critical challenge for businesses across various industries, from airlines and hotels to e-commerce platforms and subscription services. Pricing decisions must consider numerous variables, such as market conditions, competitor pricing, customer preferences, and demand elasticity.
Traditional approaches using Excel may overlook the intricate relationships between these variables, leading to suboptimal pricing decisions. AI algorithms, on the other hand, can continuously analyze real-time data on market conditions, customer behaviour, and competitor actions, enabling businesses to dynamically adjust their pricing strategies to maximize revenue and profitability.
3. Product Lifecycle Management
Understanding a product’s lifecycle is essential for businesses to make informed decisions about product development, marketing, and resource allocation. While Excel can provide basic sales data analysis, it may fail to capture the nuances of factors influencing a product’s lifecycle, such as customer feedback, market trends, competitor actions, and technological advancements.
AI algorithms can analyze data from various sources, including customer reviews, social media sentiment, market research reports, and industry trends. By identifying patterns and correlations, AI models can provide insights into a product’s lifecycle stages, enabling businesses to anticipate market shifts, optimize product portfolios, and make strategic decisions about product development, marketing, and resource allocation.
In each of these examples, the ability of AI algorithms to process and analyze vast amounts of data from multiple sources, while accounting for complex relationships and patterns, provides a significant advantage over traditional analytical tools like Excel. By leveraging the power of AI, businesses can gain a comprehensive understanding of market dynamics, customer preferences, and operational factors, enabling them to make more informed and data-driven decisions, driving innovation, and maintaining a competitive edge in their respective industries.
But what about some of the other areas of AI? If we are moving into an era of intelligent processing, what can we unlock with these new technologies? And what will help us improve Business Intelligence using Artificial Intelligence?
AI’s ability to process and analyze vast amounts of data while accounting for numerous variables is a game-changer for business intelligence (BI). However, AI’s potential in BI goes far beyond that. Here are some additional ways AI can revolutionize BI:
1. Data Preparation and Feature Engineering
One of the most time-consuming and challenging aspects of any data analysis project is data preparation and feature engineering. This involves cleaning and transforming raw data into a format suitable for analysis, as well as identifying and extracting relevant features from the data.
Feature Engineering is like coming up with different ways to sort and organize your data (which could be anything, like information about people, products, or events) so that it’s easier to understand and learn from.
An example could be with data about people’s ages. Instead of just having a number for their age, you could create a new feature called “age group” that sorts people into categories like “child,” “teenager,” “adult,” or “senior.” This new “age group” feature might help you better understand and analyze the data based on different life stages.
AI algorithms can automate and streamline this process by intelligently handling missing values, removing duplicates, and identifying and extracting relevant features from structured and unstructured data sources. This not only saves time and resources but also ensures that the analysis is based on high-quality, relevant data. Probably worth putting in a word of warning here. This shouldn’t be done without domain knowledge. We spend a great deal of time working with you to understand your processes and your datapoints to find the points that matter for you. Often there is a combination of elements that come together to make a new datapoint and this is what is generally called Feature Engineering.
2. Automated Insight Discovery
Traditional BI tools often require analysts to manually explore data and formulate hypotheses to test. AI algorithms, on the other hand, can automatically identify patterns, anomalies, and insights within the data without the need for predefined hypotheses.
Through techniques like unsupervised learning and advanced pattern recognition, AI can uncover hidden correlations, trends, and outliers that may have gone unnoticed by human analysts. This automated insight discovery can reveal unexpected opportunities, potential risks, or areas for further investigation, allowing businesses to stay ahead of the curve. This is definitely one aspect that can improve Business Intelligence using Artificial Intelligence
3. Natural Language Processing (NLP) for Data Exploration
One of the challenges of BI is effectively communicating insights to stakeholders who may not have a technical background. AI-powered natural language processing (NLP) can bridge this gap by allowing users to interact with data and analytics using natural language queries.
NLP enables users to ask questions about the data in plain language, and AI algorithms can interpret these queries, retrieve relevant information, and generate human-readable reports or visualizations. This democratizes data access and empowers non-technical users to explore and understand the insights derived from complex data analyses.
4. Continuous Learning and Adaptation
Traditional BI tools are static and require manual updates or reconfigurations to adapt to changing business environments or data patterns. AI algorithms, however, can continuously learn and adapt as new data becomes available. Try to think of new data as not only new batch data (monthly sales, or customer orders), but also as a feedback loop. What information could your users or stakeholders give to the machine to help it learn better?
Through techniques like online learning and reinforcement learning, AI models can automatically update themselves to account for new information, trends, or shifts in the business landscape. This ensures that the insights and recommendations provided by AI remain relevant and accurate, enabling businesses to stay agile and responsive to evolving market conditions. Because of automation and intelligent processing, this means that you can always stay on top of market and business intelligence, whether that is changes in competitor pricing, changes in product availability, whatever that may be. Being able to then have these changes notified helps to make all the difference. You can now have a proactive assistant that gives you the significant changes to metrics or actions you’re maybe not aware of yourself. That is a definite way to improve Business Intelligence using Artificial Intelligence.
5. Explainable AI for Transparency and Trust
One concern often raised about AI is the “black box” nature of some algorithms, where the decision-making process is opaque and difficult to interpret. However, the field of explainable AI (XAI) aims to address this challenge by making AI models more transparent and interpretable.
XAI techniques can provide explanations for the insights and recommendations generated by AI algorithms, allowing analysts and decision-makers to understand the rationale behind the AI’s outputs. This transparency fosters trust in the AI system and enables more informed decision-making based on the underlying reasoning.
By leveraging AI’s capabilities in data processing, automated insight discovery, natural language interaction, continuous learning, and explainable models, businesses can unlock the full potential of their data and gain a competitive edge through more efficient, accurate, and actionable business intelligence. You will improve Business Intelligence using Artificial Intelligence
Once a business has identified and gathered its relevant first-party data sources, such as customer data, operational metrics, financial records, and market intelligence, AI algorithms can be trained to analyze this information holistically. These algorithms can then provide predictive outputs and actionable recommendations tailored to the business’s specific goals and objectives.
For instance, a retail company could leverage AI to analyze customer purchasing patterns, inventory levels, market trends, and demographic data to optimize product assortments, pricing strategies, and targeted marketing campaigns. By accounting for numerous variables simultaneously, AI can uncover insights that may have been overlooked by traditional analytical methods, enabling the business to make more informed decisions and enhance overall performance. AI platforms can be utilised by different functions, using the same data. This helps to break down the data silo issue, but also the issue of the proliferation of ‘software that fixes one thing’.
There is a common problem in many businesses where there is a proliferation of software tools and applications that are function-specific or department-specific. This is often referred to as “software silos” or “application silos.”
Here are some key points about this issue:
1. Functional silos: Different business functions or departments often have their own dedicated software tools tailored to their specific needs. For example, marketing may use a CRM, sales may use a quoting tool, finance may use accounting software, and so on.
2. Data silos: Each of these function-specific applications typically has its own data repository or database where it stores and manages data relevant to that particular function. This creates data silos where information is fragmented across multiple systems.
3. Lack of integration: These disparate systems are often not well-integrated, making it difficult to share data and insights across functions. Data may need to be manually exported/imported or integrated through complex processes.
4. Redundant data entry: With poor integration, the same data may need to be entered into multiple systems, leading to redundancy, inefficiency, and potential data inconsistencies.
5. Limited visibility: The siloed nature of these systems limits enterprise-wide visibility into operations, performance, and key metrics, as each department only has a narrow view of their own data.
6. Difficulty with reporting/analysis: Generating reports or conducting analysis that spans multiple functions becomes extremely challenging when data is scattered across different application silos.
This proliferation of function-specific software can lead to operational inefficiencies, data quality issues, limited cross-functional collaboration, and an inability to gain a comprehensive, integrated view of the business. It can hinder data-driven decision-making and strategic planning at a business and individual level.
The solution often lies in adopting more integrated software systems, data warehousing/lake solutions, or leveraging integration platforms that can bridge the gaps between these silos. Additionally, companies may turn to business intelligence (BI) and analytics tools that can consolidate and analyze data from multiple sources to provide a unified view.
Intelligent software, that learns more about your business as you use it, can then arm you with insights that improve operations in several areas. Some call this the democratization of data, where data can be used across the organisation. It can be seen as going deeper than that. Where the needs and capabilities of each employee, in each scenario, has the support the need to deliver on their customer and business objectives. A humanised, recommendation engine, that has a feedback loop. As a productivity gain, that is a fairly compelling proposition.
Moreover, AI algorithms can continuously learn and adapt as new data becomes available, ensuring that the insights and recommendations remain relevant and accurate over time. Coupled with proactive notifications and recommended actions, AI will make BI come alive as a business operation.
Conclusion: The Future of Artificial Intelligence in Business Intelligence
In the era of big data, relying solely on traditional tools like Excel can limit a business’s ability to make informed decisions and stay competitive. Embracing AI and algorithmic data processing empowers organisations to harness the full potential of their data, unlocking deeper insights, accurate predictions, and data-driven decision-making capabilities. By leveraging the power of AI, businesses can gain a competitive edge, drive innovation, and optimise their operations for sustained success in an ever-evolving market landscape.
To experience the benefits of AI in your own organization, consider using Palm AI for Business Intelligence.
Use Palm AI for Business Intelligence.